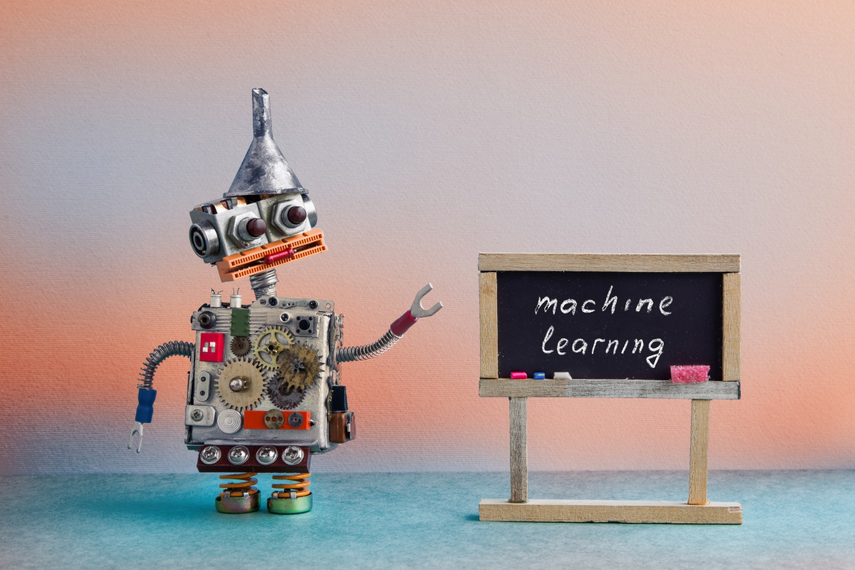
As competitive pressures mount across industries, we’re seeing a significant focused effort to drive the machine-learning agenda. Consumers now expect something in return for sharing their data, and governments are increasingly regulating the means to do so. Machine learning is seen as the leading solution for developing the next competitive advantage be that insight, process efficiency or the ability to optimise with speed and scale.
We’ve already seen that companies with machine learning at the core of their marketing will be (or already are) many times faster than their nearest rival. If we think of the first industrial revolution, production of physical product became rapid and scalable, creating unrivalled competitive advantage for companies and entrepreneurs that invested early. It's the same principle now but with scalable data analysis and insight instead of physical product.
In our recently launched whitepaper From Novelty To Necessity – Applications and Predictions for Marketing in the Machine Learning Age, we surveyed 250 of our top clients globally. Nearly half (48%) believe machine learning will allow them to automate manual tasks so they can focus on strategy, and a further 55% believe it will allow them to make better decisions through processing bigger data sets this year. Many brands are already past the hype cycle and moving into gains.
See also
AI: What to do before you even start
Time to decide which AI to talk to |
Although this presents unparalled opportunity, we must always remember that in APAC there are unique challenges implementing any new technology. With numerous markets, languages and cultures, what works for one team and one market might not for another. An in depth understanding of the technology, its application in a market context and a carefully crafted rollout strategy are essential to success.
If you’re beginning your journey, start with a small but specific business challenge, and move up from there. Here are five core principles to consider when getting started with machine learning.
1. Start with your business goals and apply machine learning where it makes sense
Every company’s goals are a little different, and there is no need to get anxious and over-apply a technology just because it’s available. Elements of a business process that have significant, regularly updated data components are great candidates for machine learning.
A fantastic example and initiative is the goal Alibaba Group and Nanyang Technology University in Singapore are setting themselves. The first research institute of Alibaba to be located outside of China, NTU and Alibaba are setting up a five-year partnership dedicated to using the power of Machine Learning and AI to research diseases such as Tuberculosis and Arthritis, potentially tapping into daily patient data to identify unseen insights and problems. If there’s data coming in, machine learning can be utilised to continually analyse, iterate and optimise.
2. Examine your customers’ behaviour and identify opportunities where machine learning can remove friction
Brands that only focus their machine-learning efforts on their internal data will miss out on one of the biggest opportunities: analyzing consumer behaviour to provide a better customer experience. Every interaction a person has with a brand provides valuable information, and this wealth of data is perfect fuel for the strengths of machine learning. In addition to optimising efficiency, machine learning is a fantastic opportunity to help brands create happier customers at scale, especially in a diverse landscape like the Asia-Pacific region.
Sharing economy businesses are built on the smart use of customer data to provide personalised experiences that unlock a service gap in the market. Machine learning is at the heart of what these companies do, but much of the time this data and the ability to process it is kept in house.
Grab just announced a groundbreaking initiative to solve one of the worst customer experiences in SEA, traffic jams! By sharing anonymised GPS data from its 500,000-vehicle fleet with government transport agencies in the Philippines and Malaysia, Grab expects to help governments plan new roads more effectively, improve traffic signal schedules and even route emergency services with greater effect. This data is only shareable thanks to Grab’s capability to analyse vast data sets at scale and unlock new customer insight.
3. Learn the technology
First, review the tools you’re currently using. You may already be paying for machine-learning features you aren’t utilising. Then, survey the landscape. For most brands and marketers, it is probably better to learn what is available instead of developing new machine learning technology. It’s a win-win solution as the more people that use the tools, the better those tools become for everyone.
We can expect a lot from Amazon in this space as it begins to branch into AI-as-a-service with services like Amazon Transcribe and Rekognition, which transcribe audio to text and analyse faces and objects in video, respectively. Amazon currently runs the AI conclave which showcases the best AI startups and talent in India, likely fuelling its Asia M&A strategy which is ramping up in the region. Hopefully this means more Amazon machine-learning applications that are built locally and scalable across the region.
4. When looking for data to fuel machine learning, don’t let perfect be the enemy of good
A marketer should start with first-party data, like site analytics, customer profiles and product feeds. The goal isn’t to achieve some mystical grand theory of data in which every single piece is connected and accurate. The goal is to tap into the wealth of data you already have and use it to fuel tools that incorporate machine learning.
5. Machine learning isn’t an instantaneous 'better' button
Once you’ve launched a machine-learning tool, it will take time to test and iterate. It’s important to give the tool time to do the “learning” part of the process before expecting performance improvements.
Bowan Spanbroek is head of product and strategy at iProspect APAC.
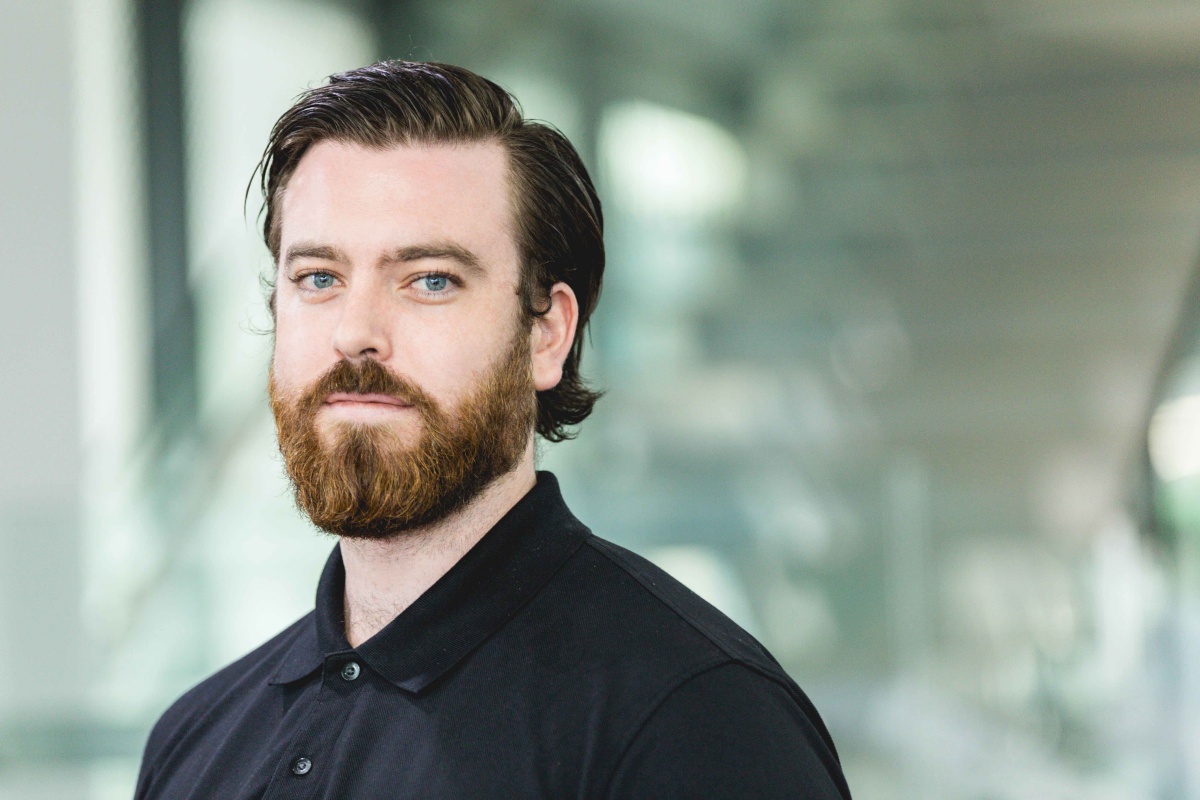