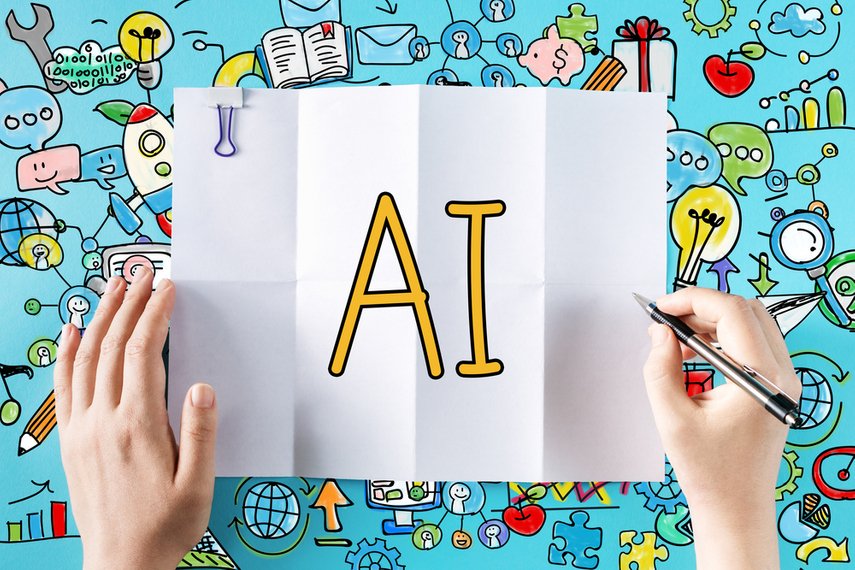
As the application of artificial intelligence (AI) is gaining momentum in media and advertising, more and more agencies are talking or writing about this subject. This trend is likely to continue in China, especially after the government recently declared its ambition to turn the country into the global leader in AI research and development. In particular, it intends to do so by raising its spending on core AI programs to US$22 billion in the next few years, up to nearly US$60 billion annually by the year 2025.
Such leadership aspirations are justified, as China already has a few success stories to exhibit. For instance, seven of the world’s 50 Smartest Companies (a list put together by the MIT Technology Review) are originally from here. Three Chinese companies alone (iFlytek at 6, Tencent at 8, and Face++ at 11) are represented in the top 11, the other eight spots all being occupied by US businesses. In general, China possesses a number of assets that makes it very well positioned in the race towards the AI pinnacle, namely thanks to the available computing power, the data generated by its 731 million netizens (as of January 2017), and the prolific publishing talent.
With all this good news about AI, however, it is easy to fall into euphoria. Various players in the advertising ecosystem, including brands, publishers, agencies, data brokers and technology vendors will want to get a piece of the pie—if not revenue-wise, then at least in terms of thought leadership and share of voice. Such zeal could easily lead to a hype similar to the one experienced in the dot-com era or, a few years later, with “big data”. As with most such episodes, everything finally ends in disillusion. In order to avoid self-deception and false hopes, it is important to have a good grasp of what the term 'AI' means, which leaves quite some room for misinterpretation.
This article defines some of the most frequently encountered expressions in the context of AI, providing examples of applications in advertising and marketing specifically. Even so, readers should be aware that the choice of a definition can be also subjective. Accordingly, no data scientist or business person will concur with these explanations. As Abraham Lincoln would say, “the subject is difficult, and good men do not agree”. Nonetheless, the present piece will hopefully contribute to disambiguating the nature of AI.
In its simplest terms, artificial intelligence is “the capability of a machine to imitate intelligent human behavior”. Thus, it involves the simulation of processes that are inherent to, or typically require, human intelligence. Examples of such processes include speech recognition, visual perception, problem solving, decision making and the translation between languages. Hence, the ultimate goal of AI is the creation of computer systems that work and react like we do.
Chatbots illustrate in a rather clear fashion how AI can be applied in marketing. These automated programs simulate human conversation through voice commands or text chats (or both). They interact with people like a human service agent would, receiving and processing messages from customers and replying to their questions or requests.
Just like in the case of the human mind, “learning” plays a fundamental role in AI. Indeed, rather than programming computers and having these slavishly execute a set of instructions meant to solve specific problems, AI is about training machines in a way they can solve tasks by using large amounts of data. This is exactly what Watson, IBM’s computer system capable of answering questions posed in natural language, does. When they created Watson (which was originally designed to compete on the US quiz show Jeopardy!), its developers trained its algorithm using 200 million pages of structured and unstructured content, including the full text of Wikipedia. Another example is AlphaGo, the computer program developed by Google DeepMind, which plays the board game Go and that beat KE Jie, the world’s No. 1, in May 2017.
This very idea of “software learned from data” (as opposed to “software written by humans”) is what makes AI inextricably connected with machine learning (ML). According to Arthur Samuel’s definition from 1959, ML is the subfield of computer science that gives “computers the ability to learn without being explicitly programmed”. It is about the creation of algorithms that can learn from and make predictions on data, in particular when the programming of explicit rules with good performance is too difficult or simply infeasible.
Typical marketing-related applications of ML include search (ranking), email filtering, or product recommendations on e-commerce websites. Publicis Media’s Content Predictor (the tool that helps prognosticate the ratings of upcoming TV dramas) is also based on machine learning. Although it is possible to “manually” create algorithms that predict the rating for a single market or a single target audience (TA), the total number of “market + TA” combinations is so large (currently more than 1,800 in total) that our development team has no other choice but to resort to machine-learning techniques.
One term that is often mentioned in connection with AI is deep learning, a subfield of machine learning “concerned with algorithms inspired by the structure and function of the brain called artificial neural networks” (ANN). Without getting too much into the technical details, the beauty of these ANNs is their ability to change (or “learn”) based on the input and output they receive and generate, respectively.
In email filtering, for example, ANNs are trained to identify spam messages by analysing example emails that have been tagged “spam” or “no spam” and using the results to identify spam in untagged emails. This is quite similar to how neurons are structured or operate in biological brains—thus the term “artificial neural network”.
Another discipline in which ANNs are also frequently employed is data mining, which may be considered as a light form of machine learning. According to the definition on Wikipedia, the goal of data mining is the “extraction of patterns and knowledge from large amounts of data”. These are then transformed into an understandable structure so that they can be exploited for business purposes.
Customer profiling, churn prediction, campaign optimisation, cross-selling, or market-basket analysis (for example, for the specification of product bundles) represent but a few of the many applications of data mining in marketing or customer relationship management (CRM). More generally, patterns that data mining can help discover include the groupings of data records (clustering), the allocation of new observations to a set of categories (classification), the identification of unusual records (anomaly detection), the establishment of dependencies (association rules), or the finding of functions between variables (regression).
Regression (or multiple linear-regression models, to be more precise) is a basic tool in econometrics, another domain that is surprisingly crucial in marketing. Although the concept, defined as “the application of statistical methods to economic data”, can be quite misleading, it is often used by data scientists to test hypotheses using real world data. One of its most prominent uses in business is marketing mix modelling (MMM). Often adopted to optimise advertising mix and promotional plans with respect to a certain KPI (sales revenue, profit, brand awareness, etc.), it utilises regression on time-series data to estimate the impact of various marketing tactics on that KPI and then forecast the impact of future sets of such tactics. The insights provided by MMM studies (including, for instance, the ROI of individual media touchpoints, the effectiveness of marketing activity, the optimal GRPs per week, and so on) make up some of the most essential elements of information for any media plan or, for that matter, marketing strategy.
Yet MMM is not the only technique that is built on statistics. Market researchers, advertisers and CRM experts often use statistics to obtain a better understanding about their customers, or about consumers in general. Statistical analysis typically comes in two flavours: a) descriptive statistics, which is about summarizing or the quantitative description of the features of a collection of information (using indexes such as the mean, standard deviation, or z-scores), and b) inferential statistics, which aims at generalising or inferring properties about a population (for example by testing hypotheses and deriving estimates).
By now, one may be tempted to think that this article is digressing from its main subject, artificial intelligence. Not so. On the contrary, all the methods described above are integral constituents of business analytics (BA), i.e. “the skills, technologies, practices for continuous iterative exploration and investigation of past business performance to gain insight and drive business planning”. In BA, statistical analysis and predictive modelling can be employed to both support human decision making and to drive fully automated decisions—similar to what AI aims to achieve. In that sense, BA and AI do have quite a few things in common.
In fact, it is recommendable to have a critical mass of structured analytics and automated processes in place before even considering the adoption of artificial intelligence. In their HBR article If Your Company Isn’t Good at Analytics, It’s Not Ready for AI, Nick Harrison and Deborah O'Neill highlight the risks of leapfrogging best practices and of directly investing in expensive startup partnerships and advanced technologies (involving black-box systems, cloud computing and open-source toolkits). Instead, the authors suggest spending time and money to build the right infrastructure and capabilities. In the context of marketing, these may include the automation of data feeds (such as from TV ratings, social media and ad tracking), the standardisation of data processes, the connection of all relevant information systems refer to a primary “source of truth” (such as providing a single view of a customer), and so on.
By the way, these recommendations are not inconsistent with the cautionary words that Maurice Lévy expressed earlier this year in Davos, when he prevised that the use—and misuse—of data would define brand success and failure in the decades to come, and that ill-conceived experiments into areas such as AI might “kill” brands.
We have been warned.
Wang Lu (王珞) is analytics director for Greater China and Olivier Maugain is general manager of analytics for Greater China at Publicis Media. |