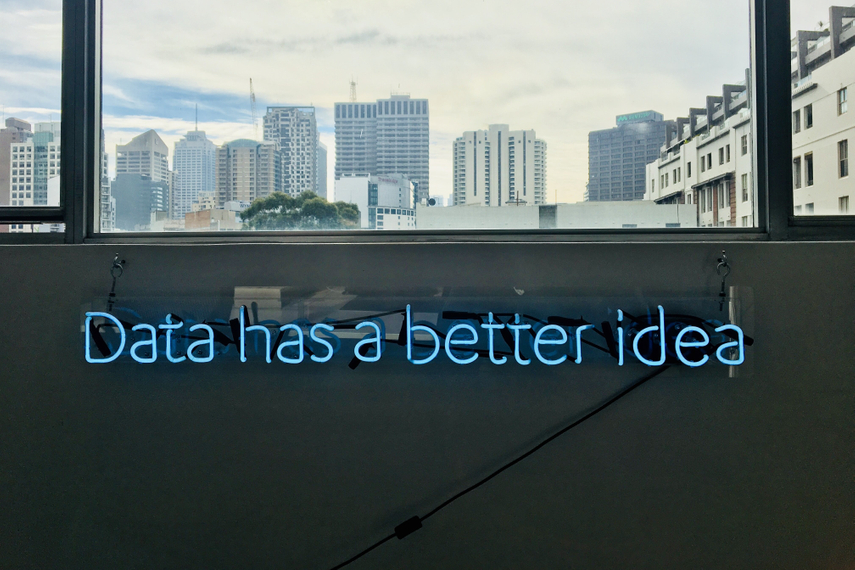
Please sign in or register
Existing users sign in here
Having trouble signing in?
Contact Customer Support at
[email protected]
or call+852 3175 1913
Bias is often perceived as a negative, but marketers can exploit it to get results quicker—so long as they understand the limitations, says Appier's chief AI scientist.
Contact Customer Support at
[email protected]
or call+852 3175 1913
Top news, insights and analysis every weekday
Sign up for Campaign Bulletins
With tech giants lobbying for AI training on copyrighted material and artists filing lawsuits to protect their work, adland faces the challenge of integrating AI while respecting copyright, originality, and the livelihoods of creators.
A PR veteran, Liu empowers the next generation in Taiwan by promoting fairness, authenticity, and industry excellence.
This Labour Day, a powerful tribute by creative agency The Friday urges us to see the workers we pass by without a second thought. But does recognition go far enough?
The subtle design refresh spans over 50 sub-brands across categories like pharmacy, groceries, and on-demand streaming under a single brand umbrella.